Putting power-hungry AI on an energy diet
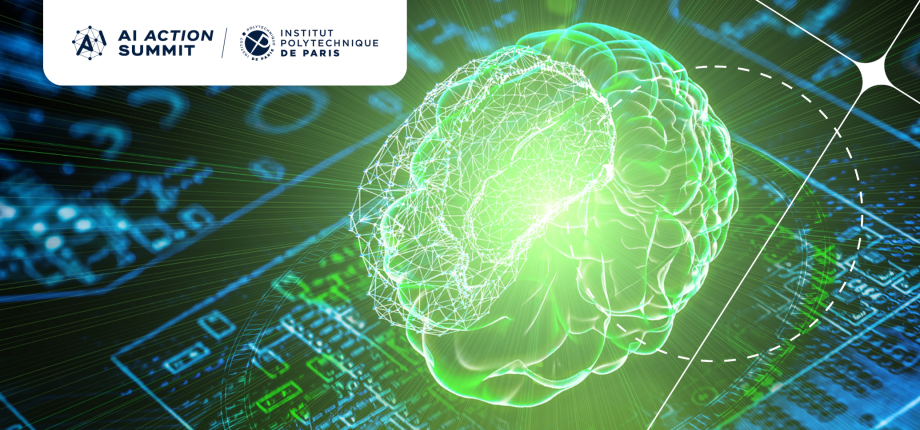
Despite some striking feats achieved over the last few years, artificial intelligence (AI) is not without flaws: the models are becoming larger and larger, requiring an increasing amount of energy to run them. In times of climate change and energy crisis, this hype on AI caught the attention of specialists like Enzo Tartaglione, an Assistant Professor at Télécom Paris member of the Multimedia Team, part of the Image, Data, Signal (IDS) department at LTCI, in who embarked upon putting the AI models on an energy diet.
“Some of these models are so large that they can’t be run locally and have to be run remotely, which poses a problem from multiple perspectives: privacy, energy consumption on the server, energy consumption in the transmission, etc,” says the scientist, who is also part of the European Lighthouse of AI for Sustainability (ELIAS), which brings together researchers in academia and practitioners in the industry “to cultivate original AI solutions that contribute to a sustainable future for our planet, foster social cohesion, and uphold individual rights”.
Greedy AI
Among the greediest is Deep Learning, which emerged about a decade ago as one of the AI champions. In Deep Learning, algorithms consist of what specialists call “artificial neural networks”, which are a subset of machine learning models (vaguely) inspired by the structure and functioning of the brain. “Algorithms’ structures are composed of layers of these neurons. The more the layers that the signal needs to travel through, the deeper the neural network is. That’s why it’s called deep learning,” says Enzo Tartaglione.
Remotely mimicking the human brain, neural networks can learn to accomplish tasks, such as image recognition, natural language processing, and speech recognition, from vast amounts of data.
“Nowadays, the race with artificial neural networks is about fitting in them more and more information, which often means having bigger models”, says the researcher. “If you want Deep Learning to learn more, you can do two things: improve the design of the learning algorithm to make it more efficient or optimize their properties; or simply make the models larger.”
Given smartphones are now more powerful than laptops ten years ago, the inflation of Deep Learning models is not entirely surprising. Taking into account technological progress, Enzo Tartaglione and his colleagues focus on designing models that consume less energy or less compact models. Which can be achieved in multiple ways.
One is called “pruning”. It consists in removing neurons, or parameters, or entire layers of neural networks that are not used for the assigned task. Another approach aims at developing models that can decide whether some intermediate computations would be useful or not. “If not, it is cut down,” sums up Enzo Tartaglione, who works on the second option.
Making smaller, more efficient models generalizable
One of the challenges is to develop efficient models that can learn, adapt and achieve similar performances with new sets of data. This generalizability of the neural network is faced with what is called spurious correlations: the neural network is relying on irrelevant patterns in input data leading to incorrect outputs. Another part of Enzo Tartaglione’s research consists in identifying those spurious correlations and correct these behaviours inside the neural networks so that the model's inductive biases don’t align with spurious patterns and exacerbate the problem of spurious correlation.
“A system that self-adapts to the user is extremely interesting, but continuously training neural networks consumes twice to three times more energy than just using them,” notes the computer scientist. Back to square one: AI models are too greedy.
Like the electric bulb was replaced with energy-saving LEDs, artificial intelligence has to undergo its own revolution. “We need to take strong actions before we over rely on an AI that requires so much energy that we reach a point of no return and completely halt. I think the research should focus on maintaining the algorithms at scale and improving their design and operating capabilities.”
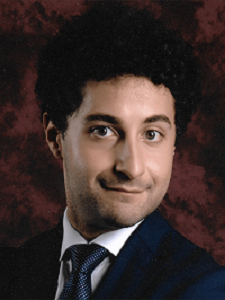
About :
Enzo Tartaglione is an Assistant Professor at Telecom Paris and he is a Hi! PARIS chair holder. He is also Member of the ELIAS Society and Associate Editor of IEEE Transactions on Neural Networks and Learning Systems. He has received the MS degree in Electronic Engineering at Politecnico di Torino in 2015, cum laude. The same year, he also received a magna cum laude MS in electrical and computer engineering at University of Illinois at Chicago. In 2016 he was also awarded the MS in Electronics by Politecnico di Milano, cum laude. In 2019, he obtained a PhD in Physics at Politecnico di Torino, cum laude, with a thesis titled “From Statistical Physics to Algorithms in Deep Neural Systems”. His principal interests include compression, sparsification, pruning and watermarking of deep neural networks, deep learning for medical imaging, privacy-aware learning, data debiasing, regularization for deep learning and neural networks growing. His expertise mainly focuses on the themes of efficient deep learning, with articles published on top conferences and journals in the field.
*LTCI : a research lab Télécom Paris, Institut Polytechnique de Paris, 91120 Palaiseau, France