The future of AI will be decentralised and collaborative
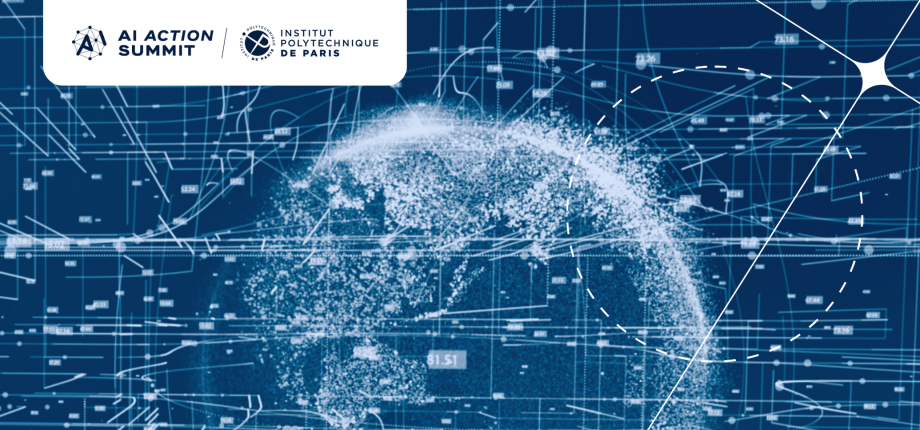
Though generative Artificial Intelligence (AI) emerged quite recently, it is already nothing like it was in its early days. “Even Large Language Models (LLMs), a type of AI program capable of recognising and generating text, among other tasks, have made huge strides since their introduction in 2022. They are more factual, hallucinate much less, and are even starting to reason,” says Éric Moulines, professor at the Center for Applied Mathematics (CMAP*) at the École Polytechnique.
As a matter of fact, generative AI delivers on all its promises. What's more, these models which, unlike traditional AI methods, seek to generate original content from training examples, can be used to solve “inverse problems”.
Restoring an electrocardiogram with generative AI
Let's imagine a worst-case scenario: a patient admitted to the emergency room undergoes an electrocardiogram on the spot. Unfortunately, the patient has moved and an electrode has come loose. The result is incomplete and the doctor cannot make a definite diagnosis. “With generative models, we learn how to restore an electrocardiogram affected by noise or for which there is missing data,” says Éric Moulines, who has been working for several years with the Institut de RYthmologie et modélisation Cardiaque (LIRYC), a university hospital institute (IHU) in Bordeaux. To do this, examples of successful electrocardiograms were provided to the model for it to learn what it looks like.
Applications using smartwatches that measure heart rate and help reconstitute electrocardiograms that can then be used by a cardiologist already exist. “We have a project to make automatic diagnoses based on pathologies, in other words to be able, for example, to reproduce electrocardiograms for which there is little data available, to supplement the data on cardiac pathologies that are poorly represented,” notes Éric Moulines.
Reversing the trend towards data centralization
Yet, every advance brings its own challenges. As for AI, the current trend is for data to be centralised in gigantic, energy-hungry servers. What if, on the contrary, AI were to operate in a decentralized way and to build on “federated learning”, so that it could serve as a tool for collaboration? This is how Éric Moulines sees the future of AI. This vision earned him in 2022, along with his colleagues Michael Jordan from the University of California at Berkeley, Christian Robert from the Université Paris Dauphine-PSL and Gareth Roberts from the University of Warwick, an ERC Synergy Grant for the On IntelligenCE And Networks (OCEAN) project.
“We believe that in the future, stakeholders in the digital sector, that is individuals and content publishers, will seek to regain control of their data, which is currently stored on servers and used to drive AI models. People will no longer so easily leave their texts and posts on social networks at the disposal of the internet giants. Data markets will develop and data used to train major AI models will be exchanged and valued. Individuals are likely to gradually become aware of the dangers of disclosing their data and will seek to ensure that their privacy is protected,” says Éric Moulines. “What's more, humans are not isolated individuals, they interact. So, how can AI be used to serve these ‘intelligent agents’ who can talk to each other, draw up contracts and set up coalitions to achieve a result that benefits everyone?” The problem is colossal.
Éric Moulines and his colleagues are therefore trying to develop decentralised AI models in which each producer of data can share it locally while keeping it private. The data would only be shared to support collective learning of the model.
Sharing data without revealing it
Let's take the medical sector as an example again. Each hospital has its own patients, medical data and diagnoses. This data is heterogeneous because MRI machines or experimental protocols, for example, differ from one establishment to another. The medical teams nevertheless may want to learn collectively, for example, a way of discriminating between cancerous cells. “One hospital may have many patients with pathology A while another has more patients with pathology B. By working collectively, hospitals can learn about both pathologies, and will be able to perform better on both instead of just one,” underlines Éric Moulines.
All that remains to be done is to find ways of motivating “intelligent agents” to share their best data, to ensure that data confidentiality and ownership are preserved, to detect free riders who would take advantage of the system without putting in their own effort, to manage heterogeneous data, etc. One of the natural forms of compensation is the ability to obtain more accurate predictions, where the prediction is no longer defined globally, but locally and contextually, stress the researchers.
“Teaching models with distributed data, that is to say data that is not centralised, is complicated. For the moment, we don't know how to do it,” admits Éric Moulines. Given the current giant leaps in the AI sector, it is very likely that this will not hold for long.
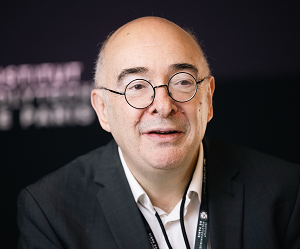
About :
Éric Moulines is professor of Statistics at the Center for Applied Mathematics at École Polytechnique. He has published over 120 articles in international journals on statistical signal processing, computational statistics, machine learning and applied probability. He has supervised more than 60 doctoral theses. He was awarded the 2010 Silver Medal by the French National Centre for Scientific Research (CNRS), the 2011 Orange Prize by the French Academy of Sciences, and the EURASIP Technical Achievement Award in 2020. He was elected to the French Academy of Sciences in 2017 in the Mechanical and Computer Sciences section.
>> Eric Moulines' personnal webpage
*CMAP : a joint research unit CNRS, Inria, École Polytechnique, Institut Polytechnique de Paris, 91120 Palaiseau, France