The digital twin, an ally for patients and doctors
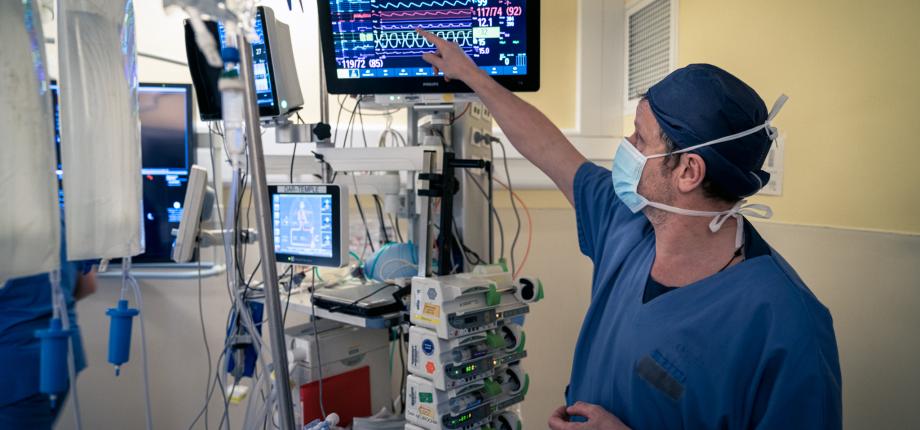
In the operating room, an anesthetist puts you to sleep. Throughout the operation, he will monitor your vitals and maintain them according to the information he has about you. But today, you're not the only patient in the operating room. Your digital twin accompanies you. “The digital twin is a software program that compiles modeling data about the patient. It can predict effects from a cause, helping the anesthetist to anticipate his or her actions,” explains Dominique Chapelle, Inria research director and member of the Inria - École Polytechnique M3DISIM research team.
In particular, the practitioner monitors your blood pressure to ensure proper blood supply to your organs. When this drops (hypoperfusion), he reacts and performs a series of actions to restore the situation. This can take time and, in some cases, leave after-effects (cognitive, cardiac, etc.). “The digital twin, on the other hand, is in predictive mode. The algorithm processes the data it already knows about you, and at the same time aggregates data on the course of the operation, producing additional information useful for monitoring anesthesia. The doctor can interrogate it at any time and have a vision of possible drifts in the next 2 or 3 minutes, in order to better adapt his actions and gain in efficiency”, adds the researcher. Such functions are also invaluable in intensive care, where patients are intubated and placed in artificial coma (the equivalent of prolonged general anesthesia). The digital twin is then able to make predictions about the evolution of their illness, enabling caregivers to carry out assessments and adapt care by limiting the number of invasive procedures.
With the help of artificial intelligence
To achieve this, the M3DISIM team is developing digital twins based on mechanistic modeling. “This involves describing cause-and-effect relationships in equations, by dissecting as many phenomena as possible. This requires aggregating data on each cause and effect involved. In biology, for example, we need to be able to observe and measure the mechanisms at play at cellular level. It's complex and time-consuming, but we sometimes use artificial intelligence to alleviate this difficulty,” explains Dominique Chapelle.
Machine learning algorithms approach this chain of cause and effect as a black box in which the mechanics at play have not been dissected and equated. It is then possible to train neural networks by basing their work on known data entering and leaving the box. This method is useful in anesthesia, for example, to determine the effects of certain drugs at the level of cardiac cells, without having all the relevant data at hand. By cross-referencing the patient's personal and functional information (cardiac parameters, how the heart works, pathology, etc.), the concentration of the injected product and its known effects, artificial intelligence predicts the pharmacological effects of the drug used.
What's more, “a mechanistic model that encounters in a patient's personal and functional data the characteristics of a pathology whose causes and effects have been equated, is capable of making consistent predictions when working with this pathology”, stresses Dominique Chapelle. This is a considerable advantage over statistical models, which can only make predictions on data relating to situations already encountered. Another advantage of mechanistic models is that, thanks to the cause-and-effect relationships they incorporate, predictions are perfectly analyzable and therefore explicable, which also makes them easier to accept.
Understanding the living world
Today, the applications of Dominique Chapelle's work focus on anesthesia and intensive care medicine, in partnership with the Lariboisière Hospital in Paris and Inserm (MASCOT laboratory). Previous research, however, has led to the creation of digital heart twins, with the aim of optimizing pacemaker implantation according to patients' particularities. However, these tools are applicable to all organs. Martin Genet, also a member of the M3DISIM team and a researcher at the LMS, is carrying out research on digital twins of the lungs to better understand certain chronic diseases such as idiopathic pulmonary fibrosis. “We need to be able to validate our models by checking that they reproduce the cause-and-effect relationship we're trying to describe. This requires a great deal of data, which is not always easy to obtain, as living organisms are not easily understood. It's a real challenge,” concludes Dominique Chapelle.
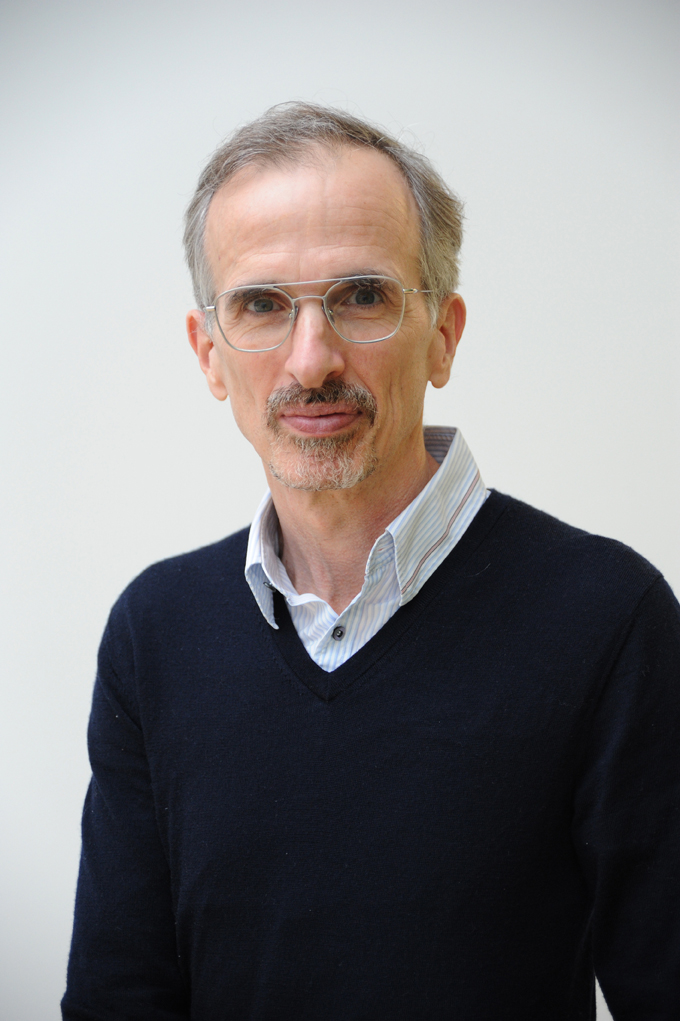
About
Dominique Chapelle is an Inria research director, researcher at the École polytechnique's Solid Mechanics Laboratory and member of the Inria - École Polytechnique M3DISIM research team. He is also scientific director of the joint AP-HP - Inria Daniel Bernoulli laboratory (Bernoulli Lab).
His work focuses on biomechanical modeling, particularly of the cardiovascular system. He fundamentally believes in the usefulness of mechanistic models and is fascinated by the idea of understanding living organisms at different scales.
His other research interests include numerical methods and mathematical analysis for structural mechanics, as well as related inverse problems.
*LMS : a joint research unit CNRS, École Polytechnique, Institut Polytechnique de Paris, 91120 Palaiseau, France