“Denoising” radar satellite images with artificial intelligence

Mounted on a satellite or an aircraft, Synthetic Aperture Radars (SAR) have revolutionized space imagery by making it possible to obtain high-resolution images of the earth, whether it is day or night, in clear or cloudy skies. The problem is that, however good they are, SAR radars are full of “noise”. “The images obtained have often a lot of fluctuations that result in altered “colours” and a kind of granularity that makes them difficult to interpret,” says Florence Tupin, a professor at the Information Processing and Communications Laboratory (LTCI*) at Télécom Paris. The researcher, who specialized in “denoising” these images with the help of artificial intelligence (AI) – more precisely with statistical learning – has developed innovative methods for “drastically improving” them.
In image processing, artificial neural networks – an adaptive AI system that teaches algorithms to process data from a large number of examples – are generally trained to obtain an image without “noise” by showing them the type of image to obtain from the acquired “noisy” image. On the other hand, Florence Tupin and her colleagues' deep learning approach makes it possible to work without a “ground truth image”. “In short, we provide the network with two noisy versions of the same scene, that is images that present the same information but with different noises, or speckle fluctuations,” says the researcher. “The network will be able to predict the part that is identical to the two images, in other words the scene without noise.”
“Denoised” images
This approach, which is called MERLIN, is based on the nature of the electro-magnetic waves measured in radar imagery, which differ from the spectral bands measured by optical sensors. These electromagnetic waves are complex numbers that can be separated into two parts, one of which corresponds to the real part and the other to the imaginary part of the complex number. That is to say, we now have two noisy images of the same scene that can be used to train a network to generate a “denoised” image, that is free of the speckle fluctuations inherent in SAR radars.
“The originality of our work is that we have shown that the images obtained from the separation of complex numbers are independent and can therefore be used to train networks to find noise-free images”, Florence Tupin sums up.
With this approach, the Télécom Paris researcher is helping to exploit radar data acquired by different sensors. Her team is helping to process data from the SWOT mission of the French space agency CNES (Centre national d'études spatiales) and NASA, whose satellite launched in 2022 is designed to study the topography of oceans and continental surface waters. Florence Tupin has also worked with start-ups, including Kanop on forest monitoring, and her team continues to disseminate for free the codes and weights of the networks developed to “facilitate their use by as many people as possible”.
Next objective: polarimetry and interferometry
“We are also developing approaches for radar tomography, an imaging technique that provides information about volumes, that is in three dimensions, which can be used to monitor biomass, in particular with ESA's Biomass mission due to be launched in 2025,” adds Florence Tupin. As with previous methods, the aim is to be able to develop “self-supervised” methods without a “ground truth image”. This has the advantage that data itself guides the network in its search for a solution.
Committed to acting as an intermediary between the space agencies that develop the instruments and the thematic researchers close to the applications, Florence Tupin and her team are now tackling radar interferometry and interferometry, which generate richer data. “It's as if instead of just having one complex number per pixel, we have several complex numbers per pixel. Therefore, we have to separate them again into two independent components”, elaborates the researcher. Is this even possible? “We already have a solution that works.”
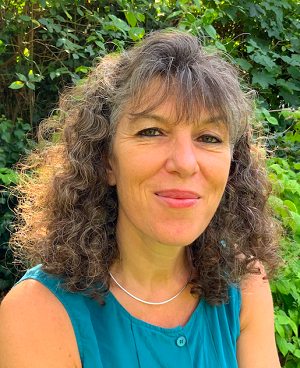
About :
Florence Tupin is researcher at Télécom Paris since 1998. Within the Information Processing and Communications Laboratory (LTCI), she works on developing image processing and analysis methods and has gradually specialised in the field of remote sensing imagery. She is working on the development of methods to make more efficient use of Earth observation images, both satellite and aerial. Her work involves a significant amount of applied mathematics, modelling and optimisation.
>> Florence Tupin's personnal webpage
*LTCI: a research lab Télécom Paris, Institut Polytechnique de Paris, 91120 Palaiseau, France