AI: towards ever more efficient image generation
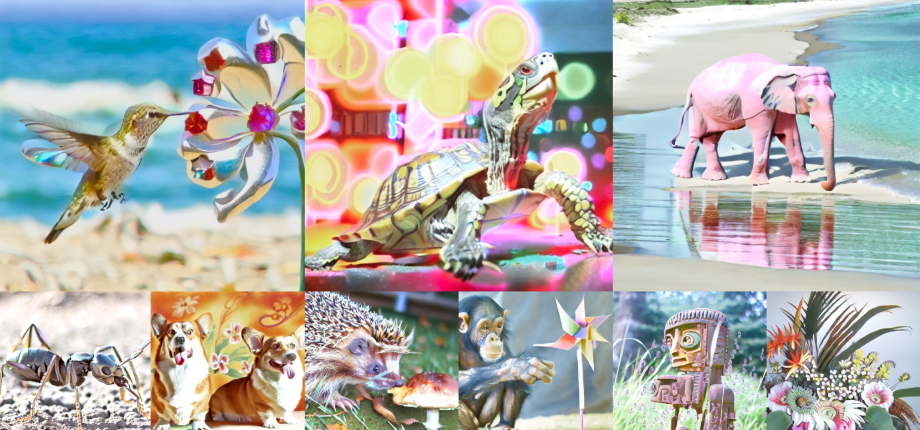
While artificial intelligence (AI) plays an increasingly important role in our lives, it is also part of Vicky Kalogeiton's daily routine. To carry out her fundamental research, the scientist deconstructs pre-existing multimodal AI models - i.e. algorithms that learn from visual, textual or even audio data - and improves them. “Firstly, we seek to understand in great detail how to use and leverage each modality, and then we develop a robust, comprehensive model for each one,” emphasizes Vicky Kalogeiton.
The researcher and her team deconstruct different types of data to teach AIs to identify key features in various situations. “For example, we trained our model to recognize the humorous elements in scenes from Pulp Fiction or Friends,” explains Vicky Kalogeiton. The system then recombines the information to generate images that consider the context (mood, tone, etc.) described by users. “We're developing third-generation generative AI that perceives and reasons, just like a human. It's no longer just about statistical data processing”.
Working with multimodal models also requires an interest in the datasets that feed them. Vicky Kalogeiton seeks to have a clear, global vision of the data used to build the knowledge of the models developed. “A model can accurately describe an unknown film sequence if it is trained with high-quality, correctly referenced data,” she explains. For example, current generative AI can recognize cinematic works 30% of the time based solely on visual information (e.g. video). A human 60%. “By way of comparison, ChatGPT, trained on articles from high-profile websites, achieves this 80% of the time. We want to give the scientific community a reliable database that enables effective research.”
A new and more efficient generative model
For image or video generation, Vicky Kalogeiton's model is trained on existing images that need to be described, tagged and labeled with text or hashtags. “This is what we call the conditions of the data,” says the researcher. There is, however, a limit to these “conditions”. If the images in the database are incorrectly filled in, then the conditions and images don't match, and part of the database is unusable. “We want to avoid this. To this end, we are proposing an AI model capable of using both labeling when it is aligned with the image and dispensing with it when it is not. A model that is both conditional and unconditional, the latter being representative of the world we live in”, explains Vicky Kalogeiton. Called Coherence Aware Diffusion, this algorithm is able to understand whether it should use the conditions associated with the data or not. This model leverages modern generative AI techniques for more efficient text-to-image generation.
“For the moment, it uses very little data, is not bulky and only handles a few tasks. It's designed for the academic world”. The challenge now is to scale up, to increase the resolution of the images generated (which means processing more data) and to increase the size of the model so that it is also suitable for industrial environments, while maintaining its efficiency. “We're still in the testing phase. We don't yet know how it will behave when scaling up”, warns the researcher. Certainly, this will make a difference to the way the model is trained, and Vicky Kalogeiton is working on latent space (editor's note: latent space is a virtual space in which complex data is encoded in a simpler, more compact, statistical form) to increase its efficiency.
Varied applications
The models developed by Vicky Kalogeiton should find applications in many fields. In video production, for example, her multimodal generative models can be used to reproduce a camera movement by generating a video. But also in the medical field, where models she and her team develop will be possible to determine in advance the risk of transplant rejection based on various visual examinations. Signs of stress on a face, electrocardiograms... are all data that can be processed by the model. The same applies to sound recordings of breathing, which in the Air Force can be used to anticipate fainting in pilots subjected to high accelerations.
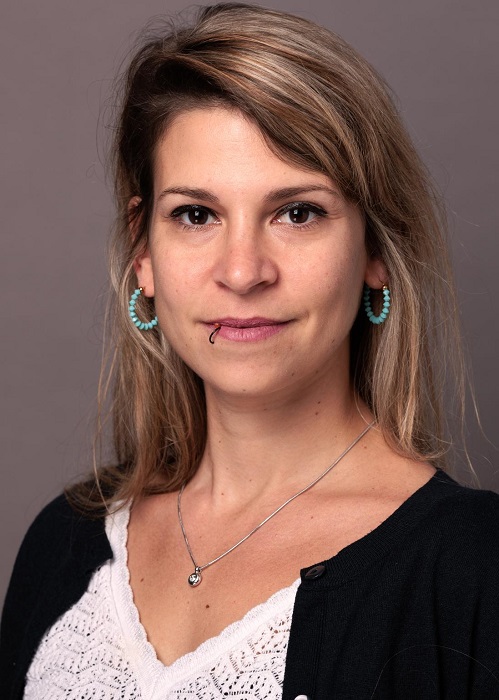
Vicky Kalogeiton is Assistant Professor of Computer Vision at the École Polytechnique and is affiliated with the VISTA team of the Computer Science Laboratory (LIX) of the École Polytechnique. She is also a member of the Ellis Unit Paris, whose aim is to foster collaborations on topics related to artificial intelligence in Paris and across Europe. Vicky Kalogeiton's research objective is to develop generalizable methods applicable to various fields, notably in multimodal generative AI, from the angle of efficiency, structured or multiple outputs, and medical applications! At École polytechnique, Vicky Kalogeiton is recognized as the leading researcher in genAI. She publishes articles in major conferences and journals specializing in computer vision (CVPR, ICCV, ECCV, T-PAMI, IJCV).
*LIX : a joint research unit CNRS, École Polytechnique, Institut Polytechnique de Paris, 91120 Palaiseau, France